Thesis: Deep Generative Shell Structure Designs – Pavlidou
-
Intro
-
Technical Aspects
Information
Primary software used | Jupyter Notebook |
Course | Thesis: Deep Generative Shell Structure Designs – Pavlidou |
Primary subject | AI & ML |
Secondary subject | Machine Learning |
Level | Beginner |
Last updated | November 27, 2024 |
Keywords |
Responsible
Teacher | |
Faculty |
Thesis: Deep Generative Shell Structure Designs – Pavlidou 0/1
Thesis: Deep Generative Shell Structure Designs – Pavlidou
A Deep Learning Framework for Optimized Shell Structures by Stella Pavlidou
In an urban context that needs to be constantly adapted to global crises, population movements, climate change and economic crises, designers and engineers strive to configure solutions that respond to multiple criteria. Within this framework, the concept of generative design is gaining more and more ground in the construction field, allowing rapid design space exploration, optimization and decision making for complex design problems. This thesis implements an experiment in a common design problem such as optimizing the topology of shell structures for structural performance, using an Artificial Intelligence Framework. To implement this experiment a novel dataset consisting of various mesh tessellations is created. The next step is to design a generative workflow that combines unsupervised and supervised learning along with a Gradient Descent Algorithm for pattern generation, structural performance estimation and optimization. A Variational Autoencoder is trained to generate new mesh tessellations and a Surrogate Model is used to predict the structural performance of the decoded designs. Finally, a Gradient Descent Algorithm searches the latent space of the Variational Autoencoder for optimum solutions. The results show that the proposed Artificial Intelligence workflow is able to generate novel and structurally better performing solutions that those existing in the training dataset. The findings of this thesis indicate that Artificial Intelligence can be successfully integrated into the concept of Generative Design to optimize shell structures.
You can find this thesis on the TU Delft Repository at ‘Deep Generative Designs’.
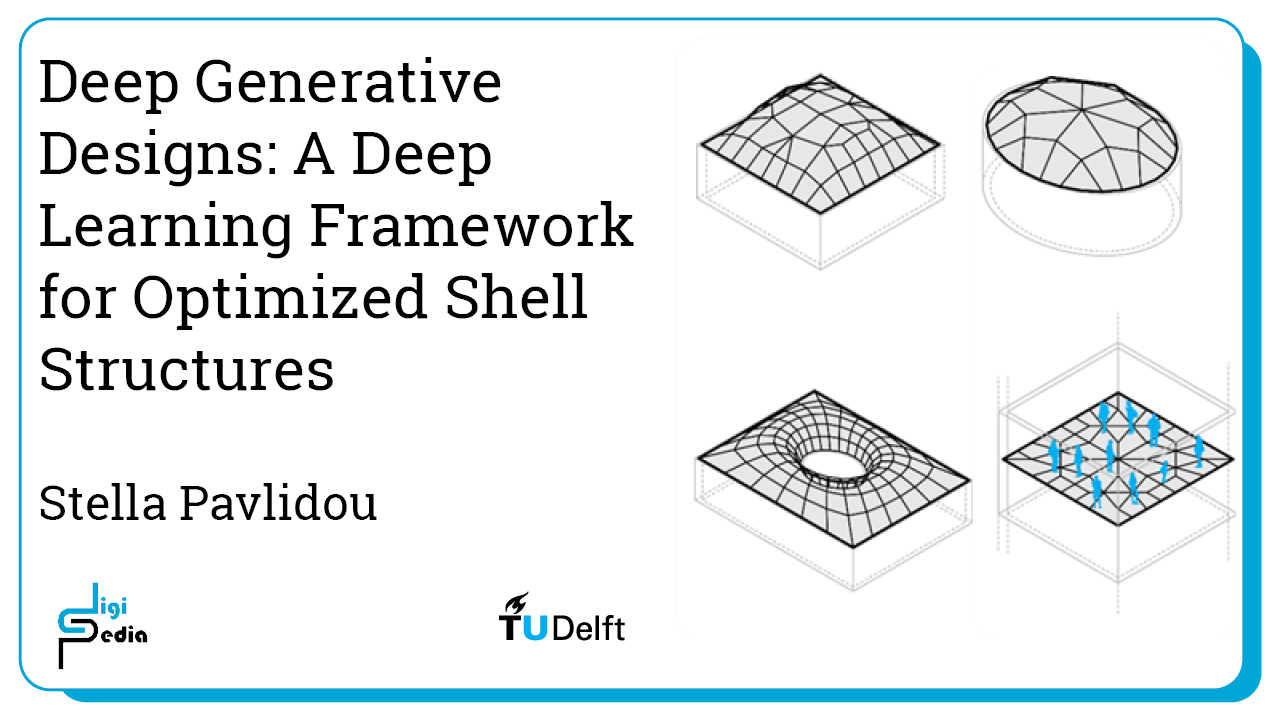
Project Information
Author(s): Stella Pavlidou
Year: 2022
Project type: Master thesis, Building Technology
Keywords: Variational Autoencoders, Surrogate Modelling, Gradient Descent
Topic tags: Generative Design, Structural Design
Thesis: Deep Generative Shell Structure Designs – Pavlidou 1/1
Technical Aspectslink copied
The objective of this thesis is to optimize the structural performance of shell structures by exploring different topology options through an AI framework. The following steps are taken:
- A new dataset is generated, consisting of several mesh tessellations created through a generative workflow..
- Next, a Variational Autoencoder (VAE) is trained using this data. To train the VAE, two methods were tested:
- The first method involves training the VAE using the coordinates of vertices as training data, but this was unsuccessful.
- The second attempt employs an adjacency matrix as the training data. This approach proved to be more successful.
- A third attempt used only a quarter of the adjacency matrix so that the input size would be smaller.
- A fourth attempt used a flattened part of the adjacency matrix , to minimize the size of the samples. This method proved to be faster.
- After training the VAE, a surrogate model was also trained to predict a performance score of decoded designs.
- A design is selected and encoded.
- The gradient of the design’s performance score with respect to its encoded vector is obtained, and a Gradient Descent Optimizer runs to optimize the initial design.
LIMITATIONS: The dataset was created using specific mesh boundaries and limited pattern exploration.. The Variational Autoencoder (VAE) was the exclusive generative model used, and the optimization process focused solely on structural performance.
SPECIAL NOTE: Code parts can be found in appendix of the thesis paper.
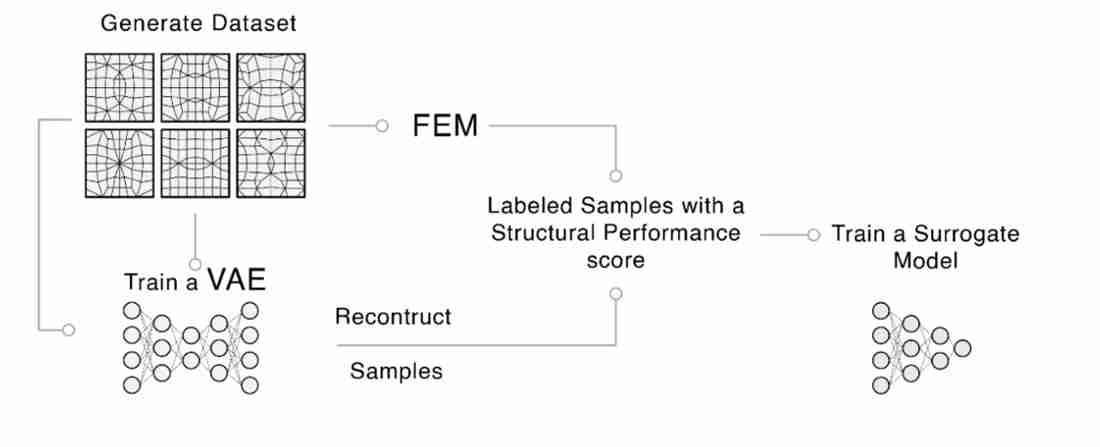
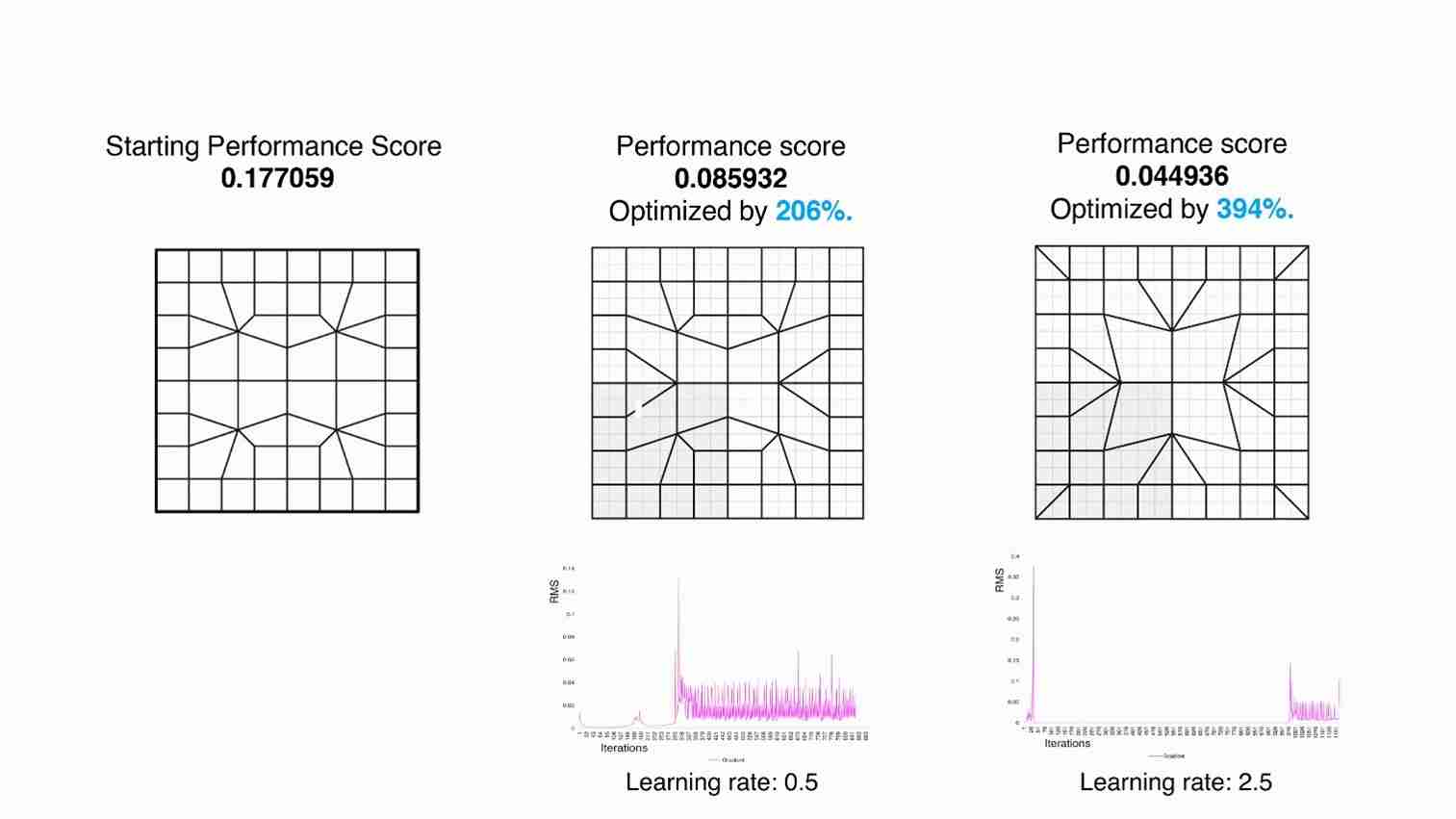
Software & Plug-ins Used
- Rhinoceros, Grasshopper, Karamba 3D for Finite Element Modelling (FEM)
- Keras (Python library) for training the VAE and the Surrogate Model
- COMPAS for the dataset generation
Write your feedback.
Write your feedback on "Thesis: Deep Generative Shell Structure Designs – Pavlidou"".
If you're providing a specific feedback to a part of the chapter, mention which part (text, image, or video) that you have specific feedback for."Thank your for your feedback.
Your feedback has been submitted successfully and is now awaiting review. We appreciate your input and will ensure it aligns with our guidelines before it’s published.