Paper: Application of Surrogate Models for Building Envelope Design Exploration and Optimization – Yang et al.
-
Intro
Information
Primary software used | Python |
Software version | 1.0 |
Course | Computational Intelligence for Integrated Design |
Primary subject | AI & ML |
Secondary subject | Machine Learning |
Level | Expert |
Last updated | N/A |
Keywords |
Responsible
Teacher | |
Faculty |
Paper: Application of Surrogate Models for Building Envelope Design Exploration and Optimization – Yang et al. 0/0
Paper: Application of Surrogate Models for Building Envelope Design Exploration and Optimization – Yang et al.
Application of Surrogate Models for Building Envelope Design Exploration and Optimization
Impacts of problem scale and sampling strategy on surrogate model accuracy: An application of surrogate-based optimization in building design
Authors: Ding Yang, Yimin Sun, Danilo di Stefano, Michela Turrin, Sevil Sariyildiz
This paper develops a workflow to train and compare different surrogate models based on different design criteria. It then allows users to use the best performing surrogate model for each criteria to optimize the building envelope designs while considering visual and energy performance.

Yang, D., Šileryte, R., D’Aquilio, A., & Turrin, M. (2016). Application of Surrogate Models for Building Envelope Design Exploration and Optimization. In R. Attar, A. Chronis, S. Hanna, & M. Turrin (Eds.),Proceedings of the Symposium on Simulation for Architecture and Urban Design (simAUD 2016) (pp. 11-14). simAUD.
Here you can find the paper Application of Surrogate Models for Building Envelope Design Exploration and Optimization
And the follow up paper Impacts of problem scale and sampling strategy on surrogate model accuracy: An application of surrogate-based optimization in building design
To create the surrogate model, the following steps are taken:
Design points are sampled by Design of Experiments (DoE): the total number of design points and their locations are defined by the user; the design points determine which numerical simulations are run. To get the most information out of a small number of simulations runs, Design of Experiments (DoE) is utilized to establish a well-distributed sampling of design points.
- Data is collected by running simulations based on the selected design points
- A surrogate model in generated through the Response Surface Methodology (RSM): Response Surface
Methodology (RSM) is a collection of mathematical and statistical methods for examining the functional connection between input and output variables. A set of algorithms is used to train multiple response surfaces for each performance indicator. The fit or quality of each obtained response surface is compared, and a final surrogate model is selected for each performance indicator
The algorithms used to create a surrogate model included “Classical meta-models” (like polynomial singular value decomposition and stepwise regression) and “Statistical-meta models” (like Shepard K-Nearest Neighbour and Kriging). To evaluate the best performing surrogate model method, 5 different surrogate models were trained. The results are then compared to find the most accurate one. The five surrogate models considered:
- Polynomial Singular Value Decomposition (SVD)
- Stepwise Regression (STEP)
- Kriging (KR)
- Shepard K-Nearest (KN)
- Radial Basis Functions (RBF).
To evaluate the applicability of RSM to the building envelope design exploration and optimization, multi-objective optimization tests were performed. Three workflows were used to evaluate RSM: two of the workflows used RSM in different manners and one did not use RSM at all. The different ways of using RSM were:
- Workflow 2: Utilizing surrogate models with an optimization algorithm to replace simulations
- Workflow 3: Using the response surface to locate the area where the optimum is likely to be, to limit down the design space in the vicinity of the optimum for further optimization
In all three situations, simulations were run. In Workflow 1, when RSM is not used, then the simulations are run during the design optimization process. In Workflow 2 and Workflow 3, the simulation is run to train the surrogate model and the surrogate model is then used during the optimization process.
The two case studies were generated with Grasshopper to test the RSM.
LIMITATIONS: An error when training the response surfaces in Case2 indicates that a larger sample size is needed for proper training and at a larger scale problem testing it may conclude in inaccuracy of the RSM.
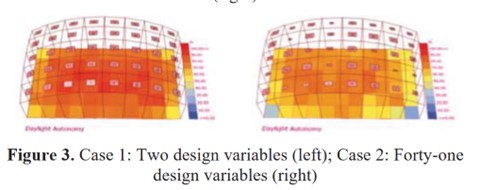
Software & Plug-Ins Used
- Rhinoceros, Grasshopper for parametric modeling
- Daysim, EnergyPlus for numeric simulations
- ModeFRONTIER for mathematical optimization
- Grasshopper plugins:
- Ladybug and Honeybee for connecting Daysim and EnergyPlus
- Customized plugin for connecting modeFRONTIER
Paper Information
- Title: Application of Surrogate Models for Building Envelope Design Exploration and Optimization
- Author(s): Ding Yang, Yimin Sun, Danilo di Stefano, Michela Turrin, Sevil Sariyildiz
- Year: 2016
- Link: https://repository.tudelft.nl/islandora/object/uuid%3Aa3fe57bc-a054-4960-88f5-0eb2f36d327b
- Type: Paper
- ML tags: Surrogate Modelling
- Topic tags: Building Energy Performance, Response Surface Methodology (RSM), Design of Experiments (DoE)
Write your feedback.
Write your feedback on "Paper: Application of Surrogate Models for Building Envelope Design Exploration and Optimization – Yang et al."".
If you're providing a specific feedback to a part of the chapter, mention which part (text, image, or video) that you have specific feedback for."Thank your for your feedback.
Your feedback has been submitted successfully and is now awaiting review. We appreciate your input and will ensure it aligns with our guidelines before it’s published.