Thesis: CNN solar panel detection – Pereira
-
Intro
-
Technical Aspects
Information
Primary software used | Jupyter Notebook |
Course | Thesis: CNN solar panel detection – Pereira |
Primary subject | AI & ML |
Secondary subject | Machine Learning |
Level | Advanced |
Last updated | November 11, 2024 |
Keywords |
Responsible
Faculty |
Thesis: CNN solar panel detection – Pereira 0/1
Thesis: CNN solar panel detection – Pereira link copied
Automated rooftop solar panel detection through Convolutional Neural Networks by Simon Pena Pereira
Transforming the global energy sector from fossil-fuel based to renewable energy sources is key to limiting global warming and efficiently achieving climate neutrality. The decentralized nature of the renewable energy system allows private households to install photovoltaic (PV) systems on their rooftops. In this context, planning an efficient grid expansion is becoming increasingly difficult. Therefore, deep learning (DL) techniques, such as convolutional neural networks (CNNs), can support collecting meta data about PV systems from aerial or satellite images, as research in the field of remote sensing has shown. However, previous research lacks the consideration of ground truth data-specific characteristics of PV panels. This thesis aims to implement a semantic segmentation model that detects PV systems in aerial imagery to emphasize the relevance of area-specific characteristics for the training data and convolutional neural network (CNN) hyperparameters. A CNN with U-Net architecture is employed to analyze the impacts of land use types, rooftop colors, near-infrared (NIR) data, and lower-resolution images on the detection rate of PV panels in aerial imagery. The results indicate that a U-Net is suitable for classifying PV panels in high-resolution aerial images (10 cm) by reaching F1-scores of up to 91.75% while demonstrating the importance of adapting the training data to area-specific ground truth data in terms of urban and architectural properties.
Here you can find the repository of the master thesis ‘Automated rooftop solar panel detection through Convolutional Neural Networks’
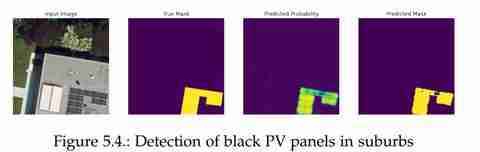
Project Information
Author(s): Simon Pena Pereira
Year: 2023
Project type: Master thesis, Geometrics
Keywords: Convolutional Neural Networks, U-Net
Topic tags: Remote Sensing, Object Detection
Thesis: CNN solar panel detection – Pereira 1/1
Technical Aspects link copied
This thesis attempts to construct a semantic segmentation model that recognizes PV systems in aerial photos to stress the importance of area-specific properties for training data and CNN hyperparameters. The thesis also investigates the effects of land use type, rooftop color, near-infrared data, and low-resolution images on detecting PV panels. The Convolutional Neural Network (CNN) uses the U-Net architecture.
The CNN computes the semantic segmentations of images. The inputs of the CNN are the aerial images which were manually labelled to indicate which regions represent PV panels.
The steps followed to train the CNN are:
- Selection of an area of interest using QGIS
- Collection of ground truth data using QGIS by manually annotating PV panels with high accuracy to be used as training data for the CNN
- Processing the ground truth data and aerial images of the same area so that the ground truth tags are overlaid unto the images
- Defining the dimensions of input patches according to the U-Net architecture and spatial resolution
- Splitting data into training, validation, and testing data sets
- Augmentation of patches
- Grouping augmented data sets into batches and defining a fixed number of iterations to run the model
- Utilization of U-Net architecture allowing training the model on few batches
The steps followed to evaluate the prediction of PV panels:
- After training a model for each area to learn area-specific properties, perform a semantic segmentation for each area respectively
- Conduct a performance evaluation of the U-Net based on the testing data set
- Visual and quantitative interpretation of the semantic segmentation and the corresponding reflectance values in the aerial images
LIMITATIONS: Due to the manual collection of ground truth data, there was a small amount of input data used for training. It is not clear what the impact of random weights is on the learning process when compared to the use of pre-trained weights.
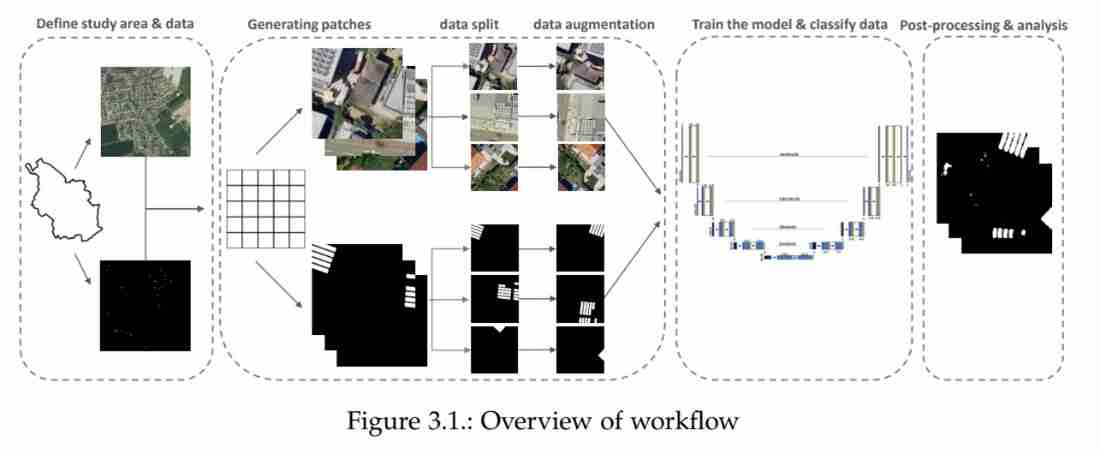
Software & Plug-ins Used
- QGIS, GDAL plugin, and R for generating and processing data
- Python using TensorFlow and Keras library for data processing and for constructing the CNN in a Google Colab-Notebook
- Google Earth Engine using JavaScript for post-processing